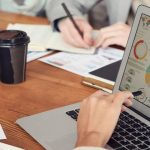
What is Business Intelligence?
July 18, 2017The terminology surrounding Big Data, Business Intelligence (BI), and data warehouses can be easily confusing. To aid in understanding, the following examples will be presented in as non-technical terms as possible:
Business Intelligence emerged in the 90s, with the goal of enhancing decision-making through the use of structured information. Structured information refers to all information within a company that is stored in a database repository, XML files or other formats that utilize a “rows and columns” structure.
By 2010, the concept of Big Data began to gain traction, particularly in relation to BI. At that time, it still mostly referred to structured data, meaning that the data was organized and easily accessible. As data volumes increased, businesses needed better ways to store and analyze this information. This led to the development of specialized types of databases, called columnar databases, such as Sybase and Vertica, which are particularly suited for data warehousing.
As it can be deduced from the name, in these databases, data is organized by columns instead of rows. This structure allows for faster data processing and is particularly useful for storing large sets of data in a way that makes analysis easier and more efficient. Moreover, it allows data compression, and quicker retrieval. Nevertheless, the term “Big Data” was not yet widely used.
So where does Big Data come from?
Big data emerged when companies like Amazon, Google, Facebook, Yahoo and others grew not only in the amount of structured data but also in non-structured information such as information in video format and social media posts. This forced the development of other tools or frameworks, which were later released as public domain (Hadoop). At this point, BIG DATA arose in full force prompting companies to adopt these new tools designed to handle large volumes of data that is not necessarily structured. Business requirements began to evolve, necessitating answers to questions derived from unconventional data sources.
As illustrated in the graph, alongside conventional sources, new sources are emerging that require different types of tools.
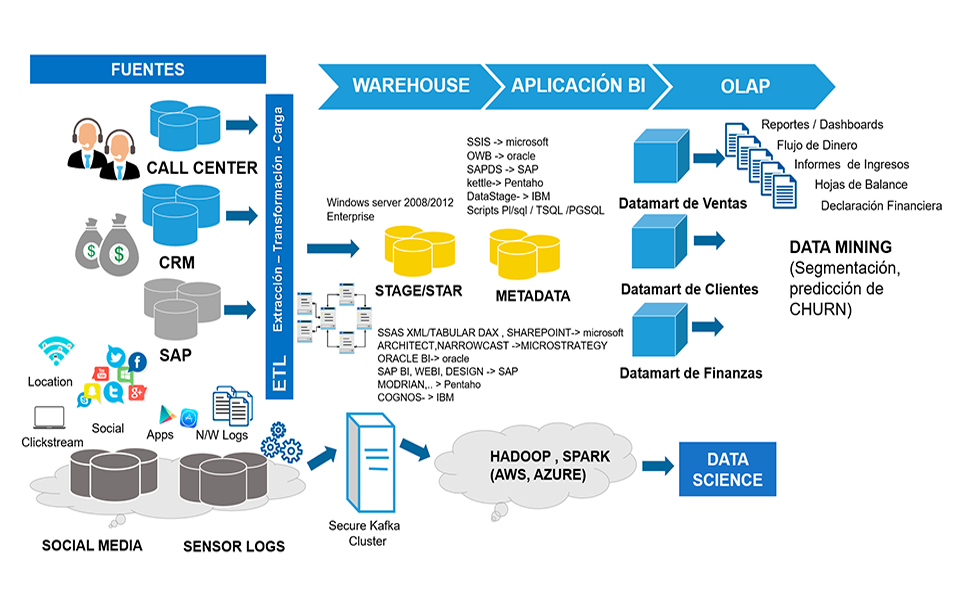
Example of customer questions
- I have my Business Intelligence platform, Data Warehouse, ETL tools (Extraction, Transformation and Loading), exploitation and analytical tools, can I replace my old platform with the new BIG DATA tools?
- My company needs to integrate different ERP and CRM systems, BPM and other internal systems. Having a lot of information makes it difficult to generate reports. Is it convenient for me to acquire a Big Data solution without the need to implement a Data Warehouse and BI?
The answers to these questions are not definite; they require asking the customer many additional business questions in order to: understand their specific needs, and provide the best alternative to their problems. The approach taken makes all the difference.
Example (generic)
Problem: The client has the need to analyze, control, and follow up, almost in real time, its customers’ response to a recently launched promotion.
- Business Intelligence Approach: You cross reference information from different CRM and ERP sources in a Data Warehouse, see the evolution of sales compared to previous months, by city, department, margins, or comparative costs, analyze and cross information from records made in the call center systems. Using data mining with historical information, it is possible to predict a possible purchase behavior in the product, anticipating possible problems.
- BIG DATA approach: Generally, uses unstructured or semi-structured information, i.e., information such as social networks, to analyze it and know what the customer thinks “sentimental analysis”, or you can go to the information that is in your call center, recordings (audio) of calls made, claims or other, to find patterns regarding the promotion, etc.
Conclusion
Big Data has significant potential for analyzing unstructured information and manage vast amounts of data, measured in Peta Bytes and Zeta Bytes. However, it is essential for this data to be integrated into a Business Intelligence or Data Warehouse tool, allowing end customers to gain insights from this complex data. Importantly, Big Data cannot replace the foundational elements created in a Data Warehouse; rather, Big Data and Business Intelligence are complementary.
For more information, you can explore this interesting additional link: (https://www.b-eye-network.com/view/17017)